Less than 1% of accounts, but almost 12% of comments: the scale of botnets in political YouTube. 39% of videos attacked by botnets.
As part of this research, a comprehensive analysis of comments on video content was conducted to identify coordinated botnet activity. The study included developing and applying a bot detection methodology, analyzing their behavioral characteristics, network connections, comment content, and temporal activity patterns.
The research aimed to understand the scale, strategies, and goals of botnet activity in the context of video content commenting.
Botnet Detection Methodology
Theoretical Foundation
Botnet detection in comments is based on a combination of several analysis methods:
- Feature-based scoring – the main method using a set of behavioral features with corresponding weights
- Anomaly detection – to identify deviations from normal user behavior
- Clustering – to group bots by similar behavioral patterns
- Network analysis – to identify connections between bots through commented videos
Mathematical model for bot detection
Volume and composition of analyzed data
As part of the study, an analysis of comments on video content collected in March 2025 was conducted. The source data had the following characteristics:
- 2148 videos
- 111 channels
- 94532 comments
Bot detection results
Application of the described methodology identified 599 bots, which accounted for almost 11000 comments left on 833 videos. Thus, despite the fact that bots made up a relatively small share of the total number of accounts (less than 1%), they generated about 11.6% of all comments and covered 38.8% of the analyzed videos.
During the analysis, it was found that some accounts belonging to channel owners demonstrate behavioral patterns similar to bots. These accounts often generate similar comments, which leads to false positives in the bot detection algorithm.
Based on manual verification, we excluded several active accounts from the bot count:
- @ZERKALOIO
- @zroblenaupolscy
- @BalaganOFF
- @viachaslau_sikora
Analysis of Detected Bot Characteristics
Distribution of bots by bot score
The visualization “Top 30 Bots by Bot Score” displays 30 accounts with the highest bot score values (rating of the probability that the account is a bot).
Key observations:
All 30 top bots have high bot score values, approximately from 0.8 to 1.0
A gradation of color intensity in the bars is observed, reflecting bot score values
Three accounts with the highest scores: @JosefOh-i8x, @Blog_Blog2, @ВячеславРязанов-63р
In the names of many accounts, characteristic patterns typical for bots are traced:
- Combination of Cyrillic names with alphanumeric suffixes
- Latin names with numeric suffixes
- First name-last name combinations with numeric/alphanumeric codes
The observed patterns in account names confirm the effectiveness of the methodology, as such template names are often used in automatic account generation.
Distribution of bots by number of comments
The visualization “Top 30 Bots by Comment Count” displays 30 bots with the highest number of comments left.
Key observations:
- Significant variance in the number of comments – from approximately 75 for the least active bots in the top 30 to more than 550 for the most active
- Two bots significantly lead others in the number of comments: @Александр-д7n1ч (~550) and @Dushman-z4f (~530)
- A sharp decrease in activity is observed after the third bot (@irishaphill7794)
- Several levels of activity among bots can be distinguished: hyper-active (>500), highly active (300-500), moderately active (200-300), low-active (100-200)
Comparative analysis with bot score distribution:
- The lists of “Top 30 Bots by Bot Score” and “Top 30 Bots by Comment Count” contain different accounts
- This indicates that the most suspicious bots by behavioral characteristics are not necessarily the most active
- The lack of overlap between top bots by score and by comment count indicates the diversity of botnets and their strategies
Bot clustering
The visualization “Bot Clusters by Comment Count and Bot Score” demonstrates the distribution of bots by two key parameters (comment count and bot score) with grouping by clusters.
Key observations:
- Most of the detected bots are concentrated in the left part of the graph (less than 100 comments)
- Bot score varies from 0.4 to 1.0, with a large concentration in the range of 0.5-0.7
- A negative correlation is observed between comment count and bot score
- Different clusters of bots with various characteristics are distinguished
- The most active bots (300-550 comments) are classified as “noise points” and have moderate bot score values (0.4-0.6)
Interpretation:
- The graph demonstrates an inverse relationship between activity and bot “obviousness”
- Different clusters represent different botnets with various strategies and goals
- Bots with high bot score indicators use more primitive tactics, while bots with high activity but lower bot scores use more advanced methods of imitating human behavior
Analysis of bot network connections
The network graph visualizes connections between detected bots, where nodes represent individual bots and edges show connections through common videos they commented on.
Key observations:
- The network demonstrates a complex, heterogeneous structure with several distinct clusters
- There is a central dense cluster with a large number of interconnections
- Several peripheral, more sparse clusters connected to the central one are visible
- The largest nodes are in the center of the graph – key bots with multiple connections
- Bots with high bot score (red nodes) are relatively evenly distributed throughout the graph
Conclusions:
- The network graph convincingly demonstrates the existence of coordinated groups of bots (botnets)
- The density of connections indicates that many bots comment on the same videos
- The central cluster represents the largest and most active botnet
- Peripheral clusters may represent separate botnets with more narrow specialization
- The scale and complexity of the network indicate a high level of organization and coordination
Analysis of Bot Comment Content
Analysis of the most frequent n-grams
The visualization “Top 3-grams in Bot Comments” displays frequency analysis of trigrams (sequences of three words) in bot comments.
Key observations:
- Two trigrams significantly exceed others in frequency of use: “zhyve belarus slava” and “belarus slava ukraine”
- Thematic clusters of trigrams are distinguished:
- Political slogans: “zhyve belarus slava”, “belarus slava ukraine”
- Cryptocurrency spam messages
- Marketing: “shou obychnoe utro”, “podderzhku shou obychnoe”
- Mentions of ethnic groups: “ugortsy finy albantsy”
- Associated with the phrase “paulya/paŭlya”: “paulya sevyarnyashcha verym”
- A surprising feature is the presence of democratic and opposition Belarusian slogans, which does not correspond to usual Russian propaganda
Interpretation:
- The presence of democratic and pro-Ukrainian slogans can be explained by:
- Bot masking strategy
- Goal of artificially enhancing discussion polarization
- Discrediting the opposition through inappropriate use of slogans
- Presence of different botnet operators with opposite political goals
Word cloud
The word cloud visualizes the frequency of individual words in bot comments.
Key observations:
- Largest words: “belarusi”, “zhyve”, “belarus”, “lukashenko”, “dzyakuj”, “vyaliki”, “rossiya/rossii”, “ukraine/ukraini”, “slava”
- Polarization of political narratives is observed: simultaneously present are pro-democratic Belarusian and Ukrainian slogans, as well as terms that can be used in pro-Russian rhetoric
- Noticeable use of several languages: Russian, Belarusian, Ukrainian
- References to historical identity (VKL, litviny) are present
Conclusions:
- The word cloud demonstrates extreme heterogeneity of political orientation in content created by bots
- The detected bots are most likely controlled by different operators with differently directed goals
- Political orientation of content should not be a factor in classifying accounts as bots
Analysis of duplicate comments
The visualization “Top Duplicate Comments” displays the most frequently repeated comments left by bots.
Key observations:
- Most common duplicates: “Dzyakuj za pratsu” (~49), “ZHYVE BELARUS” (~43), “Kamentar dlya prasoŭvannya rolika pa Yutube pavinen z…” (~40)
- Thematic categories of repeated comments are distinguished:
- Political and patriotic slogans: “ZHYVE BELARUS”, “Slava Ukraini Živie Belarus”
- Marketing and promotional: “Kamentar dlya prasoŭvannya rolika pa Yutube pavinen z…”
- Thanks and greetings: “Dzyakuj za pratsu”, “Spasibo Artyom”
- Religious and moral-ethical statements: “Dai emu Bog togo chego on zasluzhil”
- Linguistic diversity is observed: Belarusian, Russian, Ukrainian, mixed
Conclusions:
- The high frequency of identical comments unequivocally confirms the use of automated tools
- Duplicate comments serve various purposes: from political activity to content promotion
- Some comments directly indicate their marketing nature (“dlya prasoŭvannya rolika pa Yutube”)
- The use of several languages indicates an effort to reach different audiences
Temporal Analysis of Bot Activity
Activity dynamics by day
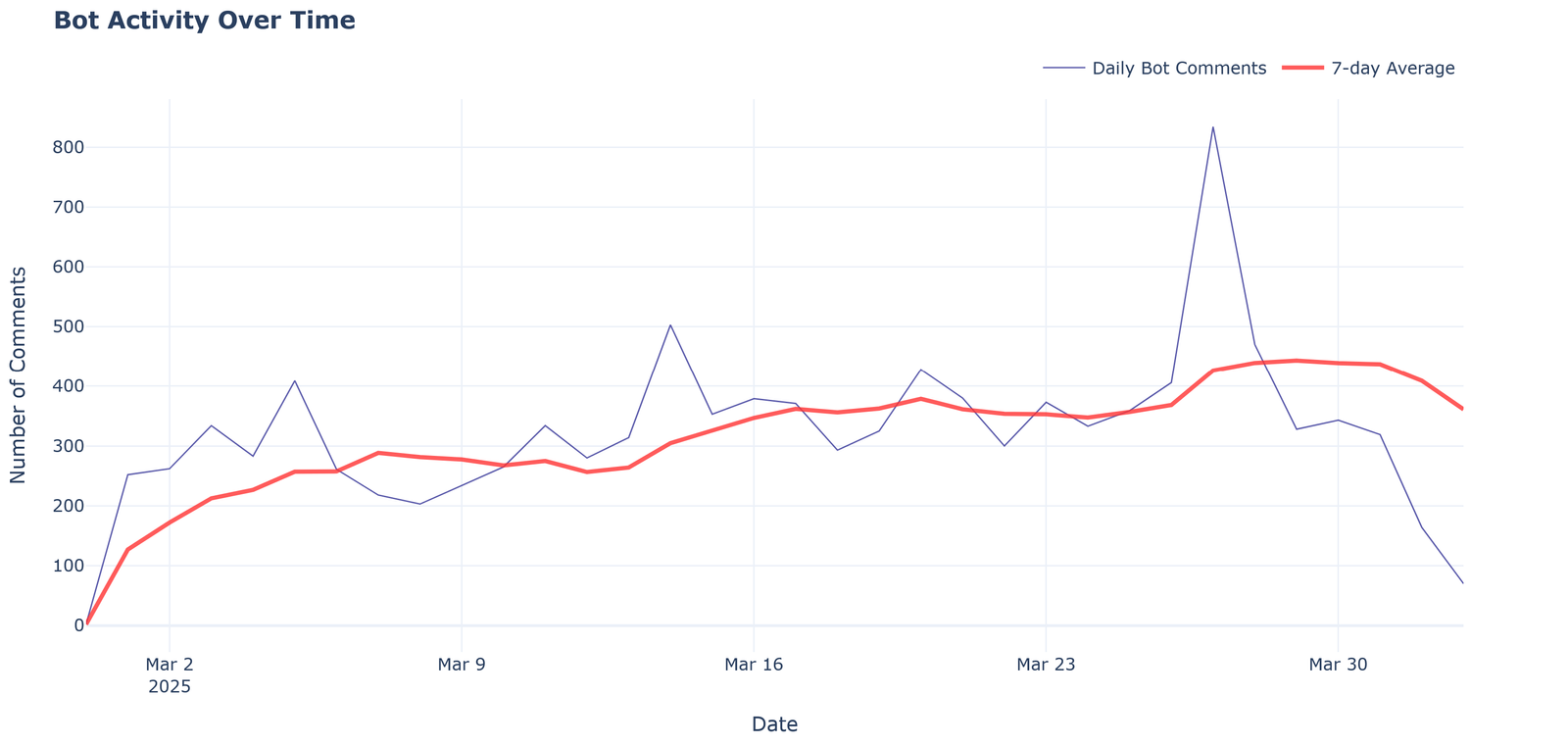
The visualization “Bot Activity Over Time” displays the dynamics of bot activity throughout March 2025.
Key observations:
- Beginning of the period (March 1): practically zero activity
- Rapid growth in the first days of March to a level of ~250-300 comments per day
- Relative stabilization in the middle of the month (300-400 comments daily)
- Sharp peak of activity on March 26-27 (~840 comments per day)
- Subsequent decline towards the end of the month
Connection with the publication of a video about Lukashenko:
- The extreme peak of activity occurs exactly on March 26, 2025 — the day of publication of the video “Lukashenko is dying before our eyes and can’t even dress himself” by the BELSAT Now channel
- The publication of this video triggered an unprecedented mobilization of botnet resources
- Elevated level of activity continued for several days after the video publication
Distribution of activity by hours and days of the week
The heat map “Bot Activity by Hour and Day” displays the distribution of bot activity by hours and days of the week.
Key observations:
- The most intense activity is observed from 6 to 10 hours and from 17 to 20 hours Greenwich time
- A clearly bipolar character of activity with two peaks (morning and evening) is traced
- Early morning hours (0-4) and late evening (22-23) show minimal activity
- Workdays show a more stable activity pattern than weekends
Analysis of connection to working day:
- Considering that the time is shown in Greenwich (UTC+0), activity peaks at 7-8 and 18-19 hours correspond to 9-11 AM and 8-10 PM in Eastern Europe (UTC+2/+3)
- These peaks clearly coincide with the beginning of the workday and evening time after work
- Activity patterns convincingly indicate that botnet management is carried out in accordance with a normal work schedule in Eastern Europe
Analysis of Target Videos
The visualization “Top 20 Videos Targeted by Bots” demonstrates videos that attracted the largest number of comments from bots.
Key observations:
- 17 out of 20 most attacked videos (85%) contain the name “Lukashenko” in the title
- The absolute leader in the number of bot comments (more than 350) is the video “Lukashenko is dying before our eyes and can’t even dress himself” from BELSAT Now
- Thematic groups of titles are distinguished:
- Health and capacity: “Lukashenko is dying before our eyes…”, “Lukashenko in RAGE”
- Threat to power: “Lukashenko has three days left”, “Lukashenko broke EVERYTHING in Belarus”
- Corruption: “Lukashenko received a bribe!”
- International relations: “Lukashenko flies to Putin for a reprimand”
- Most titles have a sensational character with clickbait elements
Hypothesis testing: The data confirms the hypothesis that using Lukashenko’s name in clickbait titles not only increases organic engagement but also provokes large-scale “bot raids.” This is especially evident for the most provocative headlines.
The visualization “Bot Activity per Video” displays the relationship between the number of unique bots commenting on a video, the total number of comments from bots, and the average number of comments per bot.
Key observations:
- Most videos attract a relatively small number of bots (up to 25 unique bots)
- The most notable outlier – the video “Lukashenko is dying before our eyes and can’t even dress himself,” which attracted 58 unique bots that left 344 comments
- Different strategies of bot usage are identified:
- Massive attack – attracting a large number of bots to one video
- Intensive attack – using a small number of bots that leave a disproportionately large number of comments
- Distributed activity – moderate number of comments from a moderate number of bots
Key Conclusions and Recommendations
General conclusions
- Scale and impact of botnets: The study revealed a significant presence of botnets in video content comments. Despite the fact that bots constituted less than 1% of accounts, they generated 11.6% of all comments and covered almost 40% of videos.
- Heterogeneity of botnets: Various types of botnets with different strategies, goals, and levels of sophistication were detected. Clustering revealed at least 7 different groups of bots with unique characteristics.
- Professional nature of operations: Temporal patterns of activity indicate that organized structures working on a regular work schedule characteristic of Eastern Europe are behind the botnets.
- Multi-directional political activity: Content analysis of comments revealed a surprising diversity of political narratives – from pro-Belarusian and pro-Ukrainian to pro-Russian. This indicates the presence of different botnet operators with different political goals.
- Content targeting: Botnets demonstrate a clear preference for videos with sensational headlines containing Lukashenko’s name,
confirming the hypothesis about the relationship between clickbait headlines and bot activity. - Coordination of actions: Significant coordination between bots is observed, especially in cases of responding to important information events, such as the publication of a video about Lukashenko’s health condition.
- Evolution of tactics: An inverse relationship between bot activity and their “obviousness” (bot score) was discovered, indicating an evolution of bot tactics to avoid detection.
Practical recommendations
- For platforms and moderators:
- Implement a multi-level bot detection system that considers both behavioral characteristics and network connections
- Pay special attention to videos with a high concentration of comments from suspicious accounts
- Pay increased attention to accounts with naming patterns typical for bots
- Track synchronized commenting activity as a sign of coordinated actions
- For content creators:
- Be aware that using clickbait headlines mentioning political figures attracts not only organic users but also bots
- Consider that high commenting metrics may be the result of botnet activity, not real audience interest
- Consider the possibility of stricter comment moderation under sensitive political content
- For researchers:
- Apply a comprehensive approach to bot detection, combining analysis of behavior, content, and network connections
- Consider that the political orientation of comments is not a reliable indicator of botnet activity
- Use temporal analysis to identify anomalous activity spikes associated with specific information events
- For users:
- Critically evaluate comments under political content, especially under videos with sensational headlines
- Pay attention to signs of potentially automated activity: similar comments, template expressions, atypical activity patterns
Conclusion
The conducted research demonstrates the complexity and multifaceted nature of the botnet activity problem in video content comments. The identified patterns indicate a high level of organization and coordination of botnets, their ability to quickly mobilize in response to significant information events, as well as the diversity of political goals of their operators.
The research results emphasize the need for continuous improvement of bot detection and countermeasure methods, taking into account the evolutionary nature of their tactics and strategies. It is important to continue monitoring and analyzing botnet activity for a better understanding of its impact on the information space and public opinion.